Hao Zhu (Stanford)- Ushering AI Agents to an Open Social World
Abstract: Unlike frontier AI models trained on static web-scale datasets, humans learn through dynamic interactions with other people and the world. This fundamental difference in learning methodology not only makes language agents less sample-efficient than humans but also introduces significant risks when these agents are deployed to interact with real humans in the real world. Building agents that can learn efficiently alongside humans remains a challenging open question.
In this presentation, I will outline three foundational approaches we’ve developed to address this challenge:
(1) Learning in social simulation (Sotopia(-π), ICLR/ACL 2024) — We demonstrate how agents can learn to navigate complex interactions with other agents under mixed and sometimes competing objectives.
(2) Learning from human decision-making (EgoNormia) — We explore methods for agents to observe and internalize social norms in physical interactions through crowd-sourced annotation.
(3) Learning to self-regulate from human feedback (AutoLibra, in prep) — We present a framework for automatically building behavior evaluation metric systems that help agents improve the policy based on human feedback.
These complementary approaches offer a path toward creating AI agents that can more effectively learn, adapt, and integrate into our open social world.
Speakers
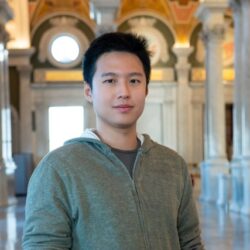
Hao Zhu
Hao Zhu is a postdoctoral researcher in the Computer Science Department at Stanford University. He finished his PhD from Carnegie Mellon University in 2024. He is interested in real world decision-makers (AI agents), human-agent interaction, robotics and embodied AI, and what AI agents tell us about human social and embodied cognition.