MS Presentation: Zhi Hong
Enabling Generalizable Scientific Named Entity
Recognition
Over the past decades, we have witnessed the explosive growth of the
hardware capabilities on computers. Machine Learning and Deep Learning
models, of which the theoretical foundations have been established
long ago, are finally computationally feasible. This does not only
affect computer science. In fact, more and more disciplines are
turning into “data sciences”, with cheaper, safer, easier data-based
simulations providing insights and guidance for traditional
experiments. These data-based methods require large of amounts of
data, especially structured data that can be easily understood and
processed by computers. Yet scientists have relied on written papers,
not digital databases, to disseminate their discoveries for several
centuries. Scientific papers are intended to be read by humans, and
most adequately convey not only discoveries, but the conditions and
methods by which those discoveries were made. Unfortunately, the
ambiguity and variability inherent in natural language makes the
automated extraction of claims from scientific papers very difficult.
Even apparently simple tasks, such as isolating reported values for
physical quantities (e.g., “the melting point of X is Y”) can be
complicated by such factors as domain-specific conventions about how
named entities (the X in the example) are referenced. Although there
are domain-specific toolkits that can handle such complications in
certain areas, a generalizable, adaptable model for scientific texts
is still lacking. In this thesis, we present our first step towards
automating this process. We have de- signed, implemented, and
evaluated models based on classifiers and neural networks for
recognizing scientific entities in free text in multiple domains.
Experiments show that our neural network model outperforms a leading
domain-specific extraction toolkit by up to 50%, as measured by F1
score, while also being easily adapted to new domains.
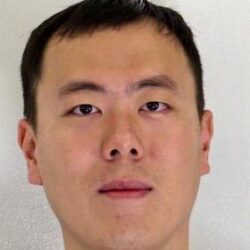
Zhi Hong
Zhi's advisor is Prof. Ian Foster